New features for scanned bioelectrical activity of motor unit in health and disease
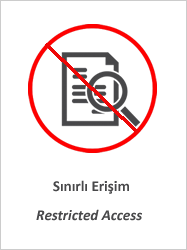
Göster/Aç
Erişim
info:eu-repo/semantics/closedAccessTarih
2018-03Yazar
Artuğ, Necdet TuğrulGöker, İmran
Bolat, Bülent
Osman, Onur
Orhan, Elif Kocasoy
Baslo, M. Barış
Üst veri
Tüm öğe kaydını gösterKünye
Artug, N. T., Goker, I., Bolat, B., Osman, O., Orhan, E. K., & Baslo, M. B. (2018). New features for scanned bioelectrical activity of motor unit in health and disease. Biomedical Signal Processing and Control, 41, 109-128. doi:10.1016/j.bspc.2017.11.011Özet
The present study aims to find new features that support the differential diagnosis of neuromuscular diseases. Scanning EMG is an experimental method developed for understanding the motor unit organization and for observing temporal and spatial characteristics of motor unit's electrical activity. A motor unit consists of a motor neuron and muscle fibers that are innervated by its motor neuron.Both simulation and biological data on neuromuscular diseases are considered in this study. Biological data were acquired from 3 patients with neurogenic involvement (2 with poliomyelitis sequela and 1 with spinal muscular atrophy), 2 patients with myopathy (1 with inflammatory myopathy and 1 with muscular dystrophy) and 4 healthy participants. Seven features are extracted by specifications of neuromuscular diseases and characteristics of EMG signals. These features are maximum amplitude, spike duration, the number of peaks, maximum amplitude x spike duration, number of peaks x spike duration, the ratio of the power outside the activity corridor to the power inside the activity corridor and the number of peaks outside of the activity corridor. The autocorrelation function of the sum of scanning EMG signals is effective in determining the activity corridor of these signals and the spike duration can be determined more easily by using the activity corridor. Wavelet transform based noise reduction and the windowing method are proposed for calculating the features correctly. By this method, spike duration and the number of peaks should be able to be calculated more precisely. It is confirmed that if the signals are filtered by a high pass filter with a cut off frequency of 2 KHz, the calculation of the number of peaks should be easier.While maximum amplitude and maximum amplitude times spike duration are found to be significant for diagnosing neurogenic diseases, other features are found to be significant for all groups by ANOVA test. It is determined that which features are more effective for differential diagnosis and the dataset that contains normal people and patients is classified using multi-layer perceptron (MLP), radial basis function network (RBF), support vector machines (SVM) and k nearest neighbor algorithm (k-NN). The best accuracy is obtained as 85% with MLP network.